Turning the table of the Rugby World Cup Rankings
08 Oct 2019
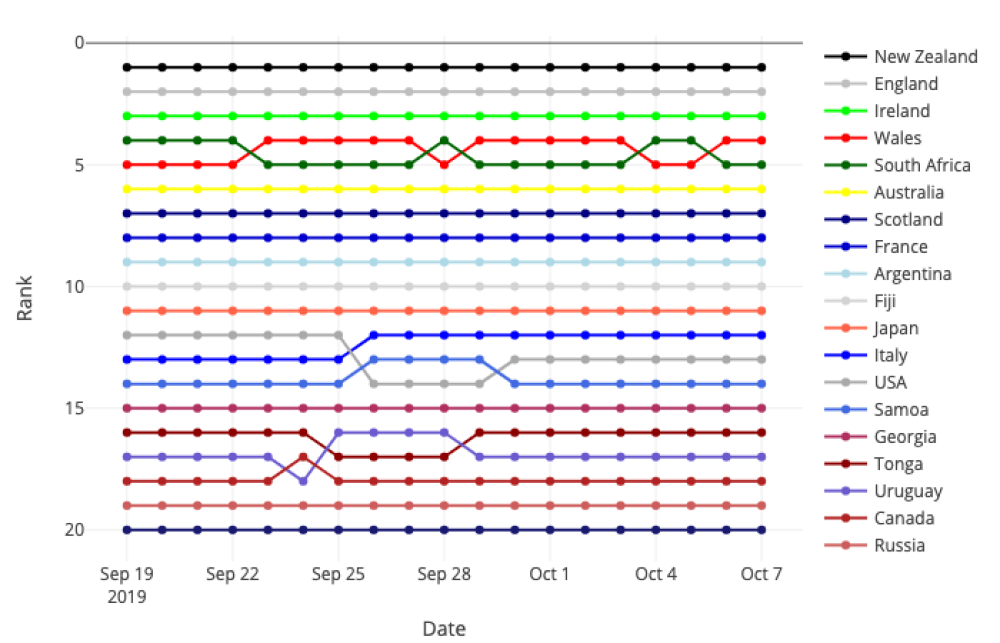
We believe ranking systems should be designed with the goal to objectively display the order of ranked subjects with as best accuracy as possible. Many official ranking systems are flawed in their mathematical approach and lack the impartiality needed to obtain a quality ranking system.
Ahead of the Rugby World Cup 2019, the Insight Data Science team wanted to address the current state of rankings system.
At the end of August Agustin Pichot, World Rugby’s Vice President called the governing body’s ranking system ‘ridiculous’[1]. Not our choice of word, but we tend to agree. While Chief Executive Brett Gosper defended the official rankings, saying that no rankings are 100% perfect.[2]
In response to this Andrew Wilson CEO of MyLife Digital, Insight’s parent company, says “We think our ranking system is pretty close. Our model is a much better reflection of projected outcomes and will become the go-to for sports journalists, industry professionals and the rugby-loving public. Our data science team has worked closely with the elite sports performance analysts to truly understand the complexities of game and built this ranking system to challenge the status quo.”
Historically, ranking strategies have commonly been based on simple win or lose outcomes. However, these outcomes do not reflect the true achievement. This is because the outcome ‘win’ does not consider the calibre of the losing opponent or the margin of victory. Without addressing these aspects, interactions between multiple teams/players are not captured.
This is a major flaw with current rankings models that needs to be addressed. Additionally, many ranking systems focus purely on one specific sport, which results in a lack of standardisation across all sports, limiting the ability to compare the athletes, or teams’, position across sport variants, such as Rugby or Football.
Our new ranking model overcomes these limitations by incorporating the missing elements, allowing for network interactions to be captured. This model is not limited to one sport variant; allowing analysts, journalists, coaches or whoever to compare team or players across different sports.
The insights produced for this Rugby World Ranking project have been created by applying our data science expertise to open sourced, public domain results data.
To test the validity of the model we have run it back over time to see the rankings generated. The simple test to compare any two ranking models is to compare the number of times each model predicted the proportion of times a higher ranked team successfully beat a lower ranked team.
As a case study we compared the performance of our model with the performance of the Official Rankings over the previous four Rugby World Cups. The overall results are displayed in the table below:
Rugby World Cup |
World Rugby Ranking Success |
Insight Ranking Success |
2003 |
90% |
92% |
2007 |
80% |
89% |
2011 |
80% |
86% |
2015 |
85% |
88% |
The results of this comparison show the Insight Ranking model has a greater success rate across all four previous world cups.
During the 2019 Rugby World Cup we shall run our ranking model alongside the official rankings. The expectation is that our model’s higher ranked teams will be victorious more often than the official ranking places them.
There is a possibility that due to random outcomes of a real tournament, that this may not be the case, but this does not discredit our model.
Our model has been created on a solid mathematical foundation and functions well with previous datasets. Any performance issues could occur from a highly surprising set of results, we will learn throughout the tournament, and if or where necessary we will make minor adjustments to improve the model.
A white paper is available for download here which contains details of the methodology, examples, analogies and future applications:
http://rugbyworldrankings.co.uk/turning-the-table-on-rugby-world-rankings/
Please login to comment.
Comments