Rubbish in-Rubbish Out: Reducing algorithmic bias
16 Apr 2021
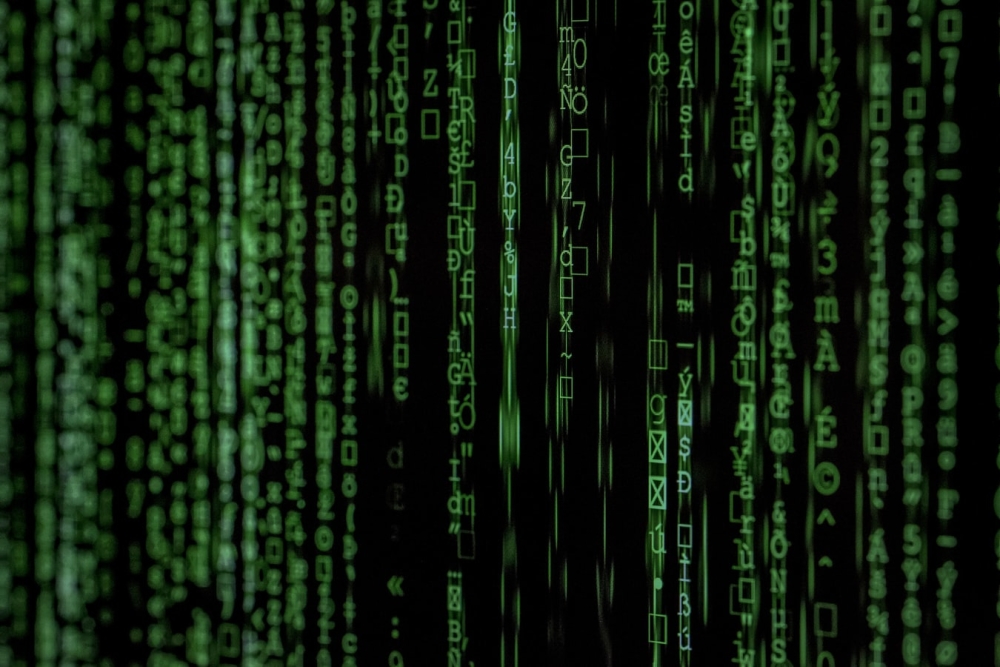
It’s unsurprising therefore that data scientists spend 60 per cent of their time cleaning and preparing data for analysis. However, 57 per cent of data scientists view this as the least enjoyable part of their job.
The fact of the matter is that data is the lifeblood of machine learning, AI, data science and analytics which is why so much time and expensive resource is devoted to ensuring that the data is as accurate as possible. The bottom line is that if the data is flawed then the resulting model will be biased, which at best will result in poor targeting and wasted marketing budget, but at worst could have severe repercussions – just ask GAFA.
A few years ago, a software engineer Jack Alciné caused a storm by pointing out to Google that their algorithm had the unsavoury tendency to classify his black friends as Gorillas. Following a public outcry for blatant racism, the giant apologised and diligently ‘fixed’ the problem. More recently, Amazon got into hot water by finding its advanced AI hiring software heavily favoured men for technical positions. Again, retraction followed the outcry. In a more newsworthy style, an unfortunate translation from Facebook accidentally got a Palestinian man arrested in Israel by mistranslating a caption he had posted on a photo of himself. Posing next to a bulldozer, the caption read ‘Attack them!’ instead of ‘Good morning!’ The man underwent police questioning for several hours until the mistake came to light.
Google didn’t go out of its way to be racist, Facebook didn’t intend to get users arrested and Amazon didn’t deliberately try to discriminate against women, but this is a result of what is known as “algorithmic bias” and it is becoming an increasingly common issue for data scientists. It is estimated that within five years it there will be more biased algorithms than there are clean ones.
Ethically understanding a model to anticipate any unintended consequences and potential bias that could impact vulnerable customers (or indeed any customer) is morally the right thing to do. It is not surprising, therefore, that data ethics are increasingly coming under scrutiny and a number of Think Tanks and organisations around the world are creating ethical frameworks to enable data science to move forwards, but in a responsible and answerable way. But it is not just a case of ethics, it is a legal requirement too.
Under GDPR companies are required to know the provenance of the data they hold and process. Furthermore, consumers have the right to know exactly what their personal information is being used for, and why decisions have been made as a result of their data. This has led to a requirement called explainability. This is the ability for a business to be to explain in human terms what is going on within the internal mechanics of a machine learning system that they use. Given that customer data is a primary source of fuel for the algorithms constructed using machine learning, organisations consequently have a legal responsibility to understand these models.
This is where data hygiene comes in. By following a regular data cleansing regime organisations can ensure that at the basest level their customer data is as accurate as possible which is a firm basis for explainability. For instance by ensuring that deceased customers, who are classified as a vulnerable group, are identified and removed or ensuring that the personal data for people that have moved house is updated it is possible to reduce the risk of bias and create a better foundation for marketing analytics and CRM initiatives. Not only that, but it reduces the time spent on data maintenance so that more time can be devoted to building more predictive models and better customer relationship driven communications that boost business outcomes. A win-win for everyone!
Please login to comment.
Comments