Natural Language Generation in Marketing
15 Mar 2018
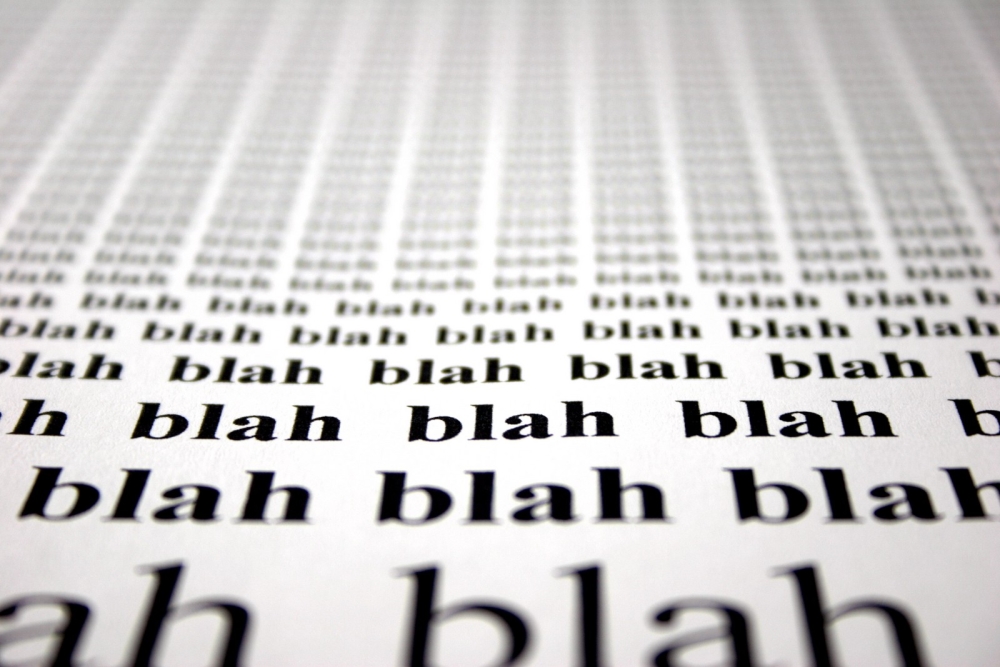
“Every time I fire a linguist, the performance of our speech recognition system goes up.”â—âFred Jelinek
A lot of the work I’ve been doing recently has been reactionary. It starts with an event, most recently a webinar on the impact of GDPR, and ends with me a long way down a wiki-hole, sure that knowing this or that article by heart will come in handy in an upcoming client meeting. I’m tempted to paraphrase Mr Jelinek’s famous (-ly misquoted) quote as ‘every time a strategist leaves, the productivity of the team goes up’. Strategy as a discipline almost exclusively contains people who are too nosy for their own good, and want to tell everyone in the office about this or that article that they simply must read because it’s great.
This month the CEO gave us a new strap-line: ‘Technology based, human driven’. This got me thinking about what an agency can do that would support this market position. We’ve seen a lot of competition in the London marketing automation sector from companies making extensive use of ‘artificial intelligence’ as their USP, and I’d like to take a deeper dive over the next couple of blogs into how that group of technologies can be separated out, and how they can support human creativity as a whole.
So what’s the difference between artificial intelligence, natural language generation, machine learning etc?
Computers, no matter how sophisticated, only view the world in terms of 1s or 0s. (Well…. With the exception of quantum computers, which I absolutely am not going to talk about here. Enjoy the wiki-hole.) Artificial intelligence, at it’s most simple, is the programming of computers to perform tasks that usually require humans, through interpreting the 0s and 1s in the same way that we interpret data from our sensory facilities. As a phrase it’s something of a misnomer, because it contains within it so many other technologies. Most of these begin with providing a computer with a tonne of data, and teaching it to recognise patterns.
In language acquisition theory, it’s generally acknowledged that a child learning a new word follows a bell curve pattern. If you have a white cat, at first the child will only use the word ‘white’ in reference to the cat. Then, as they begin to understand that ‘white’ is different to ‘cat’, they will over-apply ‘white’ to things similar to the cat, like four-legged tables. Only once they understand that ‘white’ refers specifically to the colour, and that the colour can be found elsewhere, will they be able to apply it correctly to things like snow.
The same holds true for computing processes. Snapchat, for example, graduated from learning to recognise faces, to being able to target and apply lenses using specific facial features. But, if you’re side on or there are a lot of people, the algorithm can’t get a match for the pattern. However, the more side on faces it sees, and the more flexible the algorithm becomes, the more accurate it gets.
Achieving the most accurate pattern recognition algorithm relies on machine learning, in that the programmer aims to provide the computer with the ability to learn and improve without further human intervention. For an example of this, look to Deep Mind’s development of an inter-lingua. The technology behind Google Translate has succeeded in building a language that allows it to manage multilingual zero-shot translation (it can translate without needing to be trained in a language based on reasonable pattern recognition).
Right. Now how does Natural Language Generation work?
Once pattern recognition has been achieved, and the computer is teaching itself, how does it then generate based on input? The answer to this can be found in the multi-disciplinary field of computational linguistics. It sounds like a bad joke, “what happens if you lock a mathematician, a linguist, and a computer scientist in a room together?”, but, as much as Fred Jelinek might not like it, the combination of these people yields some pretty spectacular punch lines.
If you didn’t see it, the episode of South Park titled ‘White People Renovating Homes’, is absolutely worth watching for the clip that wreaked havoc by interacting with both Alexa and Google Home devices all over the world. It was a quality prank, but the complexities of the technologies involved is incredible. Not only did those home devices recognise language coming from a digital source, they correctly interpreted what was said, generated the requested shopping list, and responded in the same natural language.
In researching how this is possible, I found many references to formal language theory and generative grammar. Noam Chomsky is the founding father of the latter field, “Observing that a seemingly infinite variety of language was available to individual human beings based on clearly finite resources and experience, he proposed a formal representation of the rules or syntax of language, called generative grammar, that could provide finiteâ—âindeed, conciseâ—âcharacterisations of such infinite languages.” (Searls in Artificial Intelligence and Molecular Biology)
The theory provided form for axiomatic reasoning with regard to cognitive processes, meaning that formal logical expressions could be used to build a mathematical theory, enabling computers to learn and generate human languages. This is the foundation for Deep Mind’s ability to work with complete phrases, comprehending grammar and syntax, rather than yielding the traditional dodgy word by word translations of old French GCSE presentations. These days you barely need to type the words in. Live translation tools like ili mean you can talk to anyone, anywhere, by ‘decoding the brainwave matrix’ with Babel Fish accuracy.
How can I use it in my marketing strategy now?
NLG increasingly gives computers the ability to turn structured data into business intelligence reports. They can develop insights based on consumer interactions, and automatically explain what course to take in response to the data. This can mean automated compliance with regulation changes, personalised content for every user, and maximum value extraction from every data set. Returning to the new strap-line, using data sets to build intelligent insights is the ‘technology base’ of any single customer view.
What is it about all this that has content marketers feeling edgy, and putting in all those extra hours to impress the boss? Any marketing professional will tell you it’s easy to produce a paragraph or two of drivel, containing buzzwords like ‘innovative’ and ‘cutting-edge’, but it is exponentially more difficult to produce excellent, brand relevant, context appropriate and personal content that captures the imagination of your audience.
The ‘human driven’ aspect comes when we evaluate the limits of NLG. There are companies building fantastic NLG subject line optimiser tools, because the computer can succeed at targeting audience motivations and raising click rates in this concise format. However, whilst generative grammar does allow axiomatic processing of much of human language, being responsive to audience motivations at scale is currently beyond the reach of the technology. It’s when you begin to write full email or landing page copy that the subtleties of human creativity continue to trounce the machine. Here, then, we find that humans are still top dog.
Please login to comment.
Comments