How to hypothesis test in any channel
02 Mar 2016
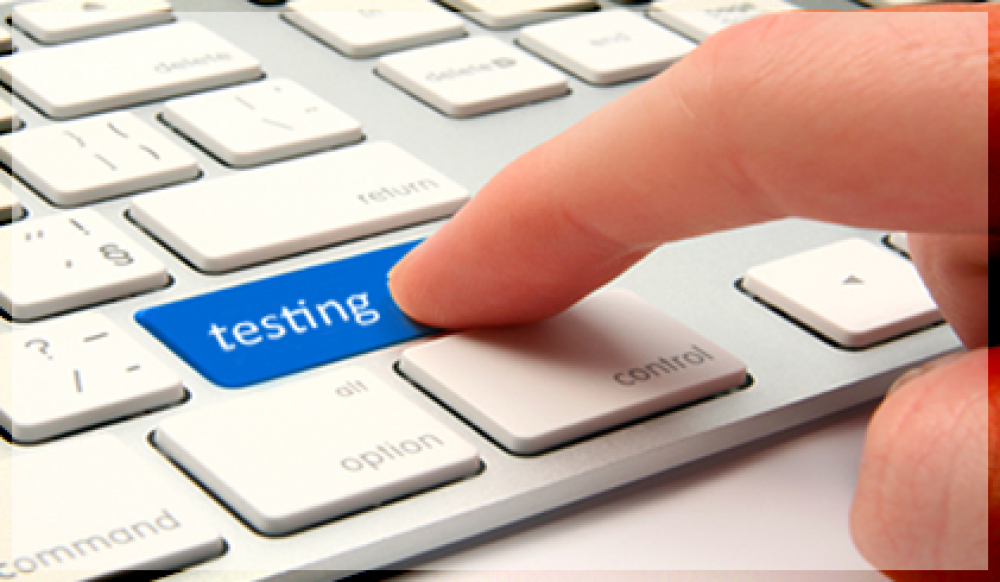
When I saw the recent results from the Marketer email tracker 2016 showing 17% of email marketers don’t conduct email testing I was a little surprised. Hypothesis testing is relatively straight-forward and yet nearly a fifth were not doing it. This was even more worrying when we found a correlation between the self-reported testing competence of marketers and reported ROI; the higher score on one, the higher the value on the other.
Now, either email marketers don’t need to test or they don’t know how. For the latter, I thought this article might be useful!
So here are the basics of what you need to know about how to test in any channel.
1.Support vs. prove
Hypothesis testing really began with one of the greatest philosophers, Karl Popper. His idea was simple; think of a hypothesis and test repeatedly, each time refining and narrowing the remit of the hypothesis. It doesn’t matter if your findings support the hypothesis, keep going because hypotheses can only be refuted.
This was eloquently described in the black swan theory. This theory was that all swans are white. You can see all the white swans in the world (supporting your hypothesis) but the hypothesis is contradicted when the first black swan is found. In other words, finding one thing that contradicts your hypothesis is more important than supporting it.
So what does this mean for marketing testing?
Let’s say you have the hypothesis that verbs in an email subject line increases opens. You conduct a test and your results support the theory. It’s important to not rest on your laurels here; keep testing. Refine your hypothesis and go again, each time the parameters of your hypothesis become narrower and narrower until you learn where the hypothesis falls down.
2.How big of a sample
One of the most frequent questions I am asked when designing a research survey is how many respondents will we need. The answer is…depends.
It depends on how big your population is, for example. The population is the total number of people that fit into your demographic or target audience. If I wanted to market to all the mothers in the US then every single mother in America would be my population. Testing them might be difficult so I might need a sample.
How big the sample of the population should be depends on the size of the population. In market research we use a measure called confidence levels. This measures how sure you can be that your result accurately represents the results of the population (if you tested them all). Generally, researchers use a 95% confident level.
The easiest way to calculate the sample size you need would be to use a sample size calculator. There are hoards online, all easy to use (for example, https://www.surveymonkey.co.uk/mp/sample-size-calculator/) .
3. What to compare against
Devising a control group is tough. This is what you compare your changed/modified group against. For example, in the email testing example I gave earlier; if one group received a subject line with verbs in it, the control group might receive a subject line with no verbs in it. It’s about knowing what to measure against.
The only advice I can give on this is that any test condition/group should only differ from the control on one variable.
For example, going back to my email example; for your hypothesis regarding verbs in subject lines, your control could not be sending no email to a group as then the two groups would differ on whether they got an email, whether there were verbs in the subject line, etc. Any differences in behaviour couldn’t only be attributed to the verb hypothesis.
Hypothesis testing, thus, takes time and means changing one thing at a time.
4.How to measure success
Success is relative. Success in regards to testing depends on what you would consider important.
You must keep in mind your margin of error and confidence level, and then ask ‘if I know what might be error and whether the result represents the population, what number outside this will I accept?’ It is a challenging question but one you must set before you test your hypothesis. Is a 2% increase in open rate a meaningful difference? That’s in your hands.
If you have any questions regarding hypothesis testing and would like my thoughts please contact me.
Please login to comment.
Comments