The data explosion: collection and refinement of consumer data
09 May 2018
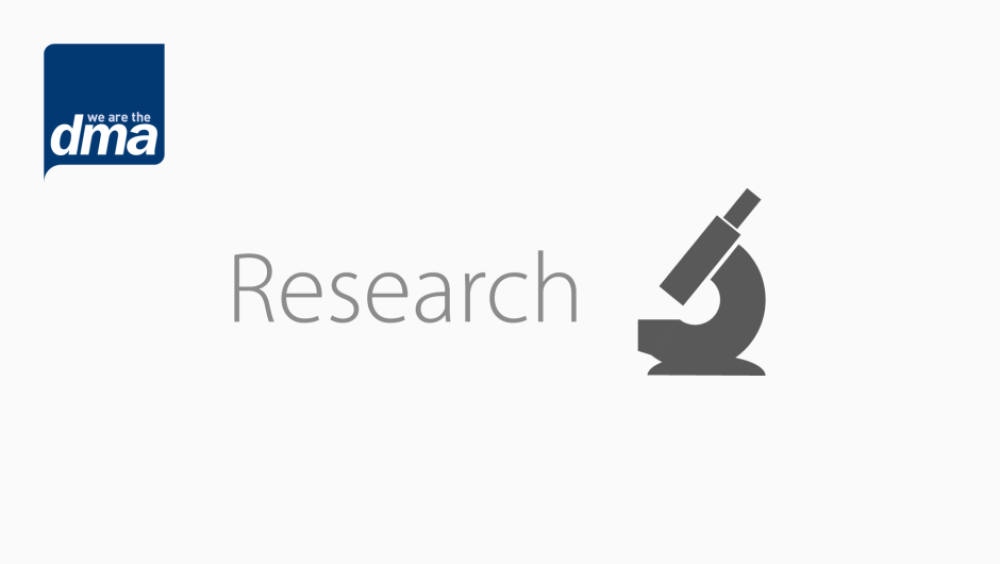
The amount of consumer data available to businesses has skyrocketed in recent years. Between 2012 and 2016, the amount of available data almost doubled each year, from 4bn to 30bn units.
Previous research from the DMA has found that consumers are more and more willing to share their data, especially where organisations are well trusted and transparent.
But how do businesses use this hoard of data to refine their business practices, improve efficiency and generate profit? The new Achieving business impact with data report from McKinsey breaks the process down in to the collection of data and refinement of data.
First, consumer data has to be collected and stored. While tech giants like Google and Amazon are collecting swathes of consumer data, the key for good data collection actually lies in selectivity, according to the authors, Niko Mohr Holger Hürtgen:
“It is simply impractical (and perhaps impossible) to collect and save every bit of the tera- and petabytes of data that will be generated every second. Defining certain requirements based on particular use cases will help ensure that only relevant data is captured”.
They also suggest a more traditional, scientific approach to data collection – with a specific hypothesis in mind – could be more useful than the often-lauded “big data” approach. Answering a specific question of interest can be more useful than searching for patterns amongst many data-points – the insight needle in the data haystack.
Then, this raw data must be refined. Here, the authors suggest a two-step “man and machine” approach – enriching the data with human insights, and pattern-discovery using machine learning. The job of the human is to clean, combine and enrich the data, before translating it into something that algorithms can easily understand.
“The combination of human hypothesis-driven input and new surprising patterns that machines reveal is the winning combination. Creating new features just helps the machine find patterns better and also helps humans better describe and act on these patterns”, the authors said.
How you process your data is up to you – using traditional analytics methods to describe the present, or predict and change the future – or using complex machine-learning algorithms.
Finally, the data must be turned in to actionable insights and these insights used to change the behaviour of your employees and shape the state of your business. This way, consumer data can become a tool to drive innovation in the marketing industry.
To read more about the research, visit: https://www.mckinsey.com/business-functions/mckinsey-analytics/our-insights/achieving-business-impact-with-data?cid=other-eml-alt-mip-mck-oth
Please login to comment.
Comments