Hereâs How Weâll Win the Cyber Security War
26 May 2016
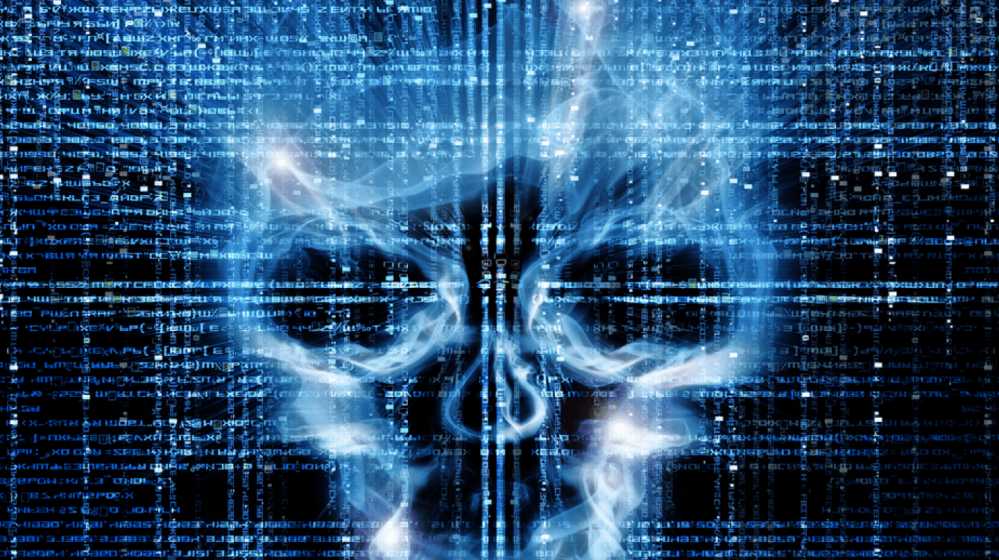
by DJ Singh
Digital Strategy Architect, Wipro Digital
Cyber attacks are increasingly making headlines with alarming regularity. They are becoming more and more difficult to detect, to the extent that many remain under the radar for long periods of time before they are even noticed. These highly sophisticated attacks are overpowering the traditional defensive mechanisms that many organizations currently have in place.
Thankfully, while hackers are becoming increasingly more cutting-edge, so too are the developments that will outpace them. Though it’s a constant struggle to remain vigilant, here’s how we’re going to get from where we currently are to where to where we should be going:
Cyber security has not yet shifted to AI systems – and they are becoming increasingly necessary
According to the Ponemon Report, it takes larger organizations up to 200 days to detect advanced threats. This is likely due to the outdated multi-layered approach many of them take to securing IT systems and data – best-of-breed point solutions that address specific security needs. For example, a solution used for monitoring employees’ web surfing is likely a point solution that has little or no integration with other security tools.
These isolated systems end up generating volumes of data, enough to confound security analysts, and more often lead to false alarms than detecting actual threats. If this didn’t sound bad enough, imagine what happens when new data sources (such as Internet of Things devices) get added to the mix!
Machine learning algorithms bring security up to speed
As depressing as the current situation sounds, there is hope in the form of recent advances in Artificial Intelligence and AI-based systems. Currently, most enterprise security architectures are designed to detect patterns (such as IP addresses of servers, unusual data access, suspicious files etc.) that rely on frequently updated ‘patches’ or ‘definitions’ to keep up with vulnerabilities. However, the latest innovation comes in the form of ‘behavioral analytics’: systems that analyze data by observing and learning to recognize unusual activity. This could be data uploads to uncommon servers, rogue servers with unusual URLs, privileged user account activity and other key ‘attack surfaces’ not usually covered by traditional security systems.
MIT’s announcement of a new AI-based Cyber Threat analysis framework gives a leg-up to this emerging approach of using machine learning models. The solution analyzes volumes of data to detect cyber security threats with a speed and accuracy current systems lack, while allowing inputs from human experts to continuously refine the system’s detection capabilities. Think of it as being like the collision-warning system newer cars have. These systems not only use pre-installed sensors and algorithms to warn the driver, but also gradually ‘learn’ the driving habits of the driver to reduce false alarms.
AI’s new battlefront: digging deeper into the Dark Web
Another developing tactic is the preemptive strike: detecting emerging threats by trying to identify them while they are still in development. The Dark Web – portions of the Web not indexed by search engines – is a fertile ground for malicious activities. Real-time data analysis of the Dark Web could involve scanning for new malware releases, or observing the activities of hackers operating in anonymity.
Cyber criminals invariably trade spoils of their loot on the Dark Web much before their victims notice the loss. Cyber security threat-detection systems can gain the upper hand by proactively monitoring for new threats. Illicit activities, such as trading of credit card information, ransom-ware tools etc. can be tracked to identify new threats and determine the patterns of attacks being planned.
Our adversaries have historically been a step ahead in finding a way to beat the system, often relying on the weakest link (users). Detecting such vulnerabilities has been an ongoing struggle, one that requires constant tactical improvements.
Automation offers strength in numbers
The effectiveness of AI-based systems in detecting threats is yet to be fully determined. AI-based systems demand new skills and a steep learning curve from IT security professionals who were trained to work with traditional security procedures, tools and technologies. Early adopters may face challenges in integrating all the data sources these systems need. The data science models used in such systems are complex and need to be continuously adjusted by hard-to-find experts. Until the system has finished learning the patterns it aims to detect, it is unlikely to completely solve the concerns and issues cyber security experts face.
While solutions such as MIT’s are no silver bullet, early adopters stand to gain in the long run from the increased level of automation this solution offers. Automation allows experts to focus on a shorter set of alerts, while in the process also making the AI system smarter. Networks of such systems have the potential to share their intelligence and learn quickly enough to outsmart any oncoming invasion.
Originally published on IT Pro Portal.
Please login to comment.
Comments