DATA MINING: 5 THINGS YOUâVE ALWAYS WONDERED ABOUT PREDICTIVE MODELS
11 Apr 2017
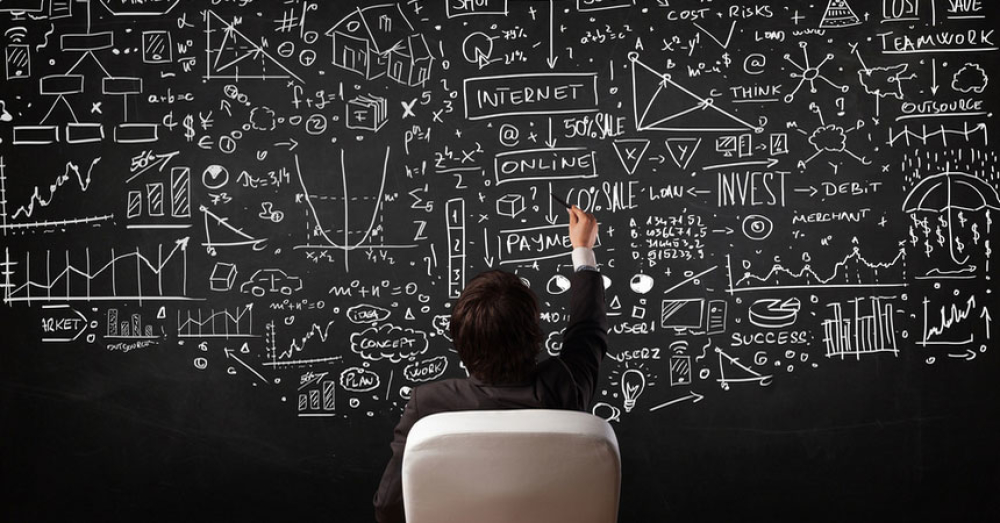
âWhat is a predictive model?
In the context of digital marketing, a model can be seen as a mathematical function into which we input certain data that describes an individual that we want to establish a prediction for. The outcome is a score for that particular individual in response to a specific classification request.
For example: we can imagine a model of clickers that addresses a question such as “What is the probability of that internet user clicking on a link in my next car dealership newsletter?” by providing a value of between 0 (lower probability) and 1 (higher probability), thus determining their ‘likelihood of clicking’.
What questions can a model address?
The first essential step before implementing the model is to determine the question you want an answer to. From the moment you open dialogue between your brand and your customers (the individuals interacting with your brand), a model can help to forecast future behaviour on whatever communication channel is required, whether online or offline.
Using predictive models, we can, for instance, ask ourselves very direct questions regarding email communications: who are the future openers and clickers of my message? By targeting these two groups in your next communication, you’ll be able to maximise your campaigns’ open and click rates.
In addition, predictive models allow you to identify future churners (individuals who are about to unsubscribe). Excluding them when targeting your campaigns will maintain the lifetime value of your customers (their value over your entire relationship with them) and maintain a good deliverability rate.
In terms of display, predictive models can answer questions about campaign performance by identifying future clickers on a banner ad, future purchasing or conversion intent, future online (on your website) and offline (point of sale) buyers, products they are likely to purchase, etc.
Predictive models can also be used to ask questions about ‘life moments’ – which of the individuals in my customer base are about to buy or renovate their home?
To conclude, we can say that a predictive model answers questions about forecasting future behaviour and can be used to discover previously unknown characteristics of an individual by detecting twin profiles, also known as ‘look-alikes’.
What data do we use to build a model?
This is the second step to take before implementing the model – determine the data sources that we can use to answer the identified question.
The data collected via each communication channel can be as follows:
- Email: open rates, click rates and complaints about past email campaigns
- Web or display: past website browsing data, ad banner impressions and clicks
- Offline: in-store purchase history
Generally speaking, we can use the target’s CRM data such as socio-demographic characteristics and declared information. We can also enrich our knowledge of individuals in the database using open data provided by institutions or third-party data.
Naturally, the wider the range of sources we use, the more opportunities the predictive model will have to detect points of interest in response to the question. As such, a truly multi-channel approach will be more powerful than an approach that is limited to a single channel.
How do we build a model?
Building a predictive model is referred to as ‘learning’. Multiple methods are available when building a model. One well-known and efficient approach within the digital marketing industry is known as ‘supervised learning’. This is a specific type of machine learning that is particularly suited to classification questions (yes/no questions).
A supervised learning model is trained by example: we provide the system with a series of positive examples (those that have the desired characteristic) and negative ones (those that don’t have the desired characteristic). These examples are provided with a tag (positive or negative) alongside a collection of data describing them. Step by step, this will help the model to refine and clarify its learning.
This approach is intuitively similar to the one we would use to teach someone to recognise a type of object. For instance, if we wanted to teach someone to recognise apples, we would show them several apples, of all shapes, sizes, colours and varieties, telling the person that they are apples (positive example). We would also show our pupil all sorts of fruits that are not apples, telling them that this is the case (negative example). Little by little, the person would build up a mental model of what an apple is.
In the same way, a computer using a supervised learning algorithm is capable of applying a similar approach and of using the training population in order to extract general characteristics that are related to the classification question.
From this description, it’s clear that the volume of data available is an essential factor: a model can only work effectively if we provide enough examples. Although negative examples are easy to find, positive ones are usually less common. For instance, average click rates rarely approach the 50% mark that we would ideally want when building a model.
How does a model work?
Once a predictive model is built, the intention is to use it with new, previously unknown data and individuals. Given an individual who wasn’t used to train the model, based on all or some of the individual’s characteristics, a model will be able to give that person an affinity score with the positive class it was trained with. This approach comes down to formulating an inference on the nature of the new individual.
In the same way, to return to our previous example, someone who has built a mental model of an apple would be able to say whether a new unknown fruit is an apple or not.
To take an example from digital marketing: using behaviour during past campaigns of an individual who is included in an email database, alongside other available information on that person, we can build a model to predict churn that will be able to provide a score (between 0 and 1) measuring the risk of churn (unsubscribing) in the near future.
This score can quickly be determined, with low latency, for any new individual (who was previously unknown and not used for the learning process) entering the database, and for all known and existing individuals who have recently carried out an action and modified their own data, such as opening a newsletter (thus creating a new state for the individual).
About the author: Julien Budynek has worked in computing since the end of the 20th century, and has worked in artificial intelligence, predictive analytics and data science over the course of his career.
Please login to comment.
Comments