Why âpredictiveâ is the wrong way to think about engaging with people
25 Jan 2017
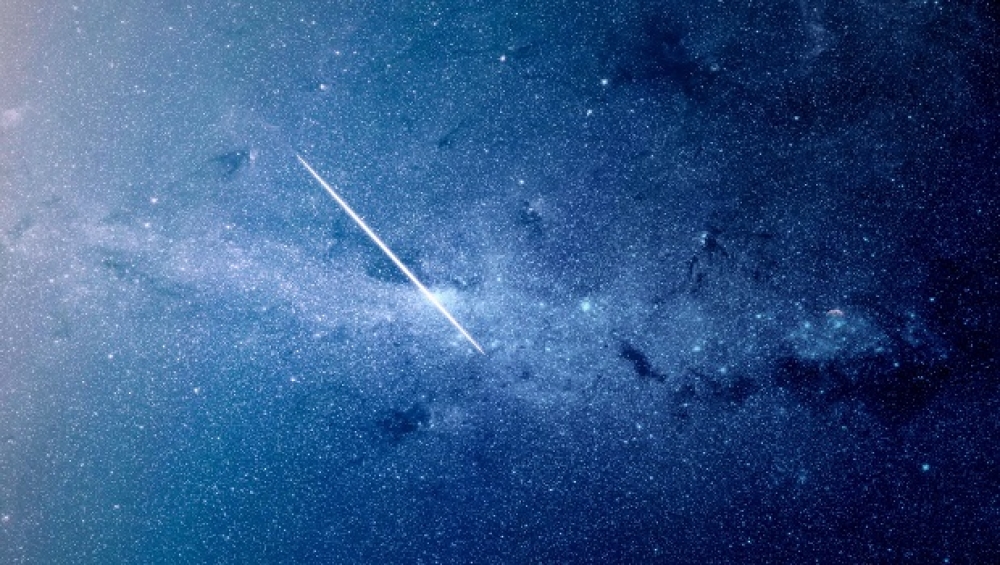
January is a month of many promises: resolutions, grand gestures; over-zealous sales emails from brands professing to be your best friend; marketing predictions from industry thought-leaders (read some smart predictions-about-predictions at the end of this article).
The great thing about promises is that they’re real and meaningful, until - or unless - they’re not fulfilled. With some already broken, and some held onto with hope, I’d like to propose a few ways to think about customer engagement which focus on proven behaviour and certainty, rather than presumptives and best guesses.
-
Know who you’re speaking to - on an individual level
We spend so much time thinking about how direct mail works, how display campaigns work, how search works; that we’ve been distracted from what matters: how people work.
As a person, it’s nice to be recognised straight away, regardless of context - and paradoxically, technology really makes this possible. The past five years of marketing tech have meant that brands can talk to an audience with more or less the same attributes as their own; but that’s not good enough anymore. We don’t want to be treated as approximations of ourselves. Instead, we want our interactions to be fully realised - and this means marketers need to realise the ability to own, carefully cross-pollinate, and accurately match data from all platforms to build a bigger, multi-dimensional picture of each customer. Then, once you don’t just think it’s them - you know it’s them, you can talk to them wherever they are, via a single customer profile.
2. It’s time to be useful, so listen to what your audience needs
There’s a lot to be said for listening, but in our land of perpetual push messaging we seem to forget the value of insights and more crucially - using those insights to be more helpful and relevant to those who matter. Take a loyal account holder at a bank, for instance: with her single profile, you already know she has had a savings and student account with you for years - and has been browsing for mortgages recently. Offer her something she really needs, when she needs it; a mortgage meeting, and then a rate she can’t refuse. Why not add real service? Share a list of her nearest branches via your app when she searches for an ATM, or offer the right consultation opportunity via the right channel - such as virtual assistant for a help-to-buy scheme, so she can better manage her money.
Remember that although what matters most to people in life is invisible, people are strongly indicating their intent online, surrounding your brand, and beyond it. This opens up the opportunity - and hands brands the responsibility - to add true value.
3. Don’t be afraid to try, fail, and learn
Of course, the true nature of predicting isn’t just throwing a dart in the dark; it’s usually an educated guess based on previous experiences or existing information. What I’d challenge everyone to consider is that the promise of predictive technologies can make us complacent about learning as people. We need to dip our toes in the water, and recalibrate what we think we know now more than ever before. People are fickle, so with the tooling in place to make sure we can zoom in on customer data, segment and tweak on a granular level, our engagement with customers needs to become more of a subtle - and nuanced - Q&A.
For example Tom, who browses flights to Paris for Valentine’s Day, on a popular comparison site. He compares offers from KLM, British Airways & Easyjet.
Previously, he’s flown with KLM. Because KLM match 2nd party data with their own databases; they know that this is the same Tom who opts for vegetarian meals and extra legroom:
KLM: re-targeted ads via Display channels to enjoy an exclusive offer on that flight you’re interested in?
Tom: doesn’t click ads. Wants to be in control. Continues to compare offers.
KLM: listens. Removes Tom from Display campaign selection.
KLM: sends Tom an email a month before departure based on plane fill-rate and previous booking habits - ‘We’ve got the lowest price for two tickets to Paris, with your favourite in-flight meal and front row seats thrown in for good measure.’
Tom: ‘yes please’ - adds flight to basket but gets distracted and abandons booking. A week later, he downloads the KLM app.
KLM: reminds Tom via the app, his next-best-channel, with a helpful deep-link to where he left the booking journey: ‘oh, and here’s a list of discount vouchers for restaurants & attractions in Paris once you’ve booked. ’
Of course Tom books the flight.
Professor Philip Tetlock, based at the University of Pennsylvania, famously did a 20-year study of political predictions involving more than 280 experts, and found that on balance their rate of accuracy was little better than chance. According to Tetlock, there are real limits on how predictable the future can be.
So as people ourselves, I urge us all to think about qualifying the intent of fellow people - even if your martech is automating and scaling these conversations. Artificial Intelligence and its machine learning algorithms won’t work without humans feeding in, and managing the smart business rules it builds on. Going forward this means acting on curiosity to try out new ways to reach people; listening and delivering a value or a service to someone you know, rather than pushing and shouting a scripted story to a distant passerby.
We love a paradox. Leading on from our anti-predictions rant, read some smart predictions from marketing leaders for this year.
Please login to comment.
Comments