The clustering myth: why modern retailers need to move on in 2018
20 Dec 2017
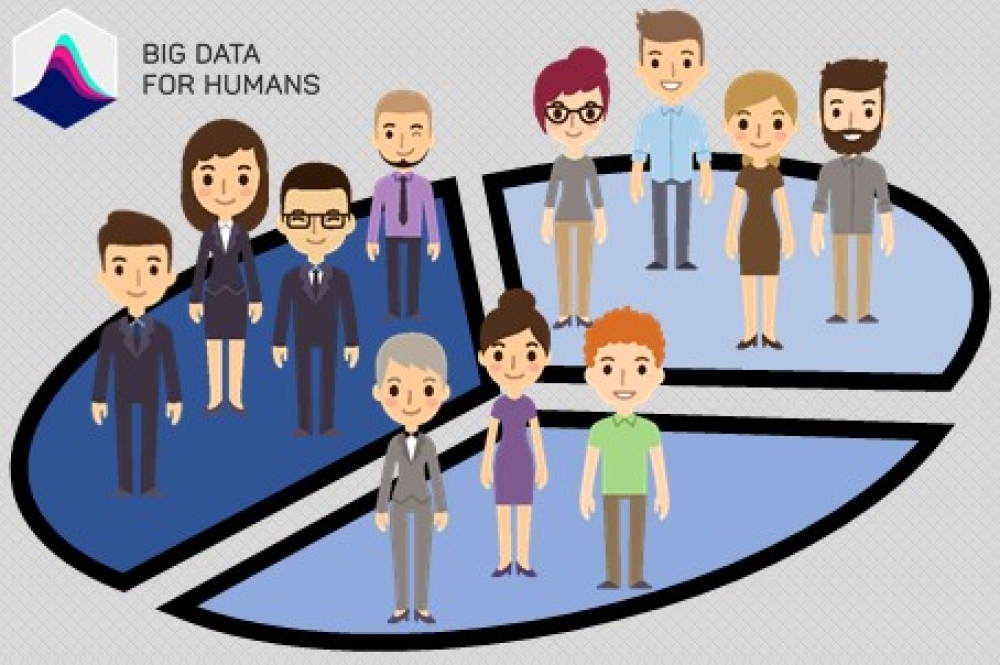
Customers’ purchasing habits are changing faster than they have in decades. So have you ever wondered why so many retail businesses still try to understand and segment their customers using legacy clustering methods that were first developed 25 years ago?
Customers have changed, and so have the rules
When customer segmentation methodologies for retail were first developed, the rule was that these segments had to be identifiable, accessible, actionable, differentiable, substantial and stable. Through my experience working for global retail and travel businesses, the first 4 still apply. But with significant advances in data science, technology and computing power, ‘substantial’ and ‘stable’ no longer make the cut when it comes to modern day ROI generation. Here’s why.
Myth: Segments have to be substantial - it’s not cost-effective to target small groups of customers
Fact: Customers expect personalisation at every touchpoint. To be successful, businesses have to be able to target small and niche groups of customers, as well as individuals
Myth: Segments have to be stable for a long enough time so that the strategy can be defined and then actioned against groups of customers who aren’t changing all the time
Fact: Customers aren’t stable and therefore your models shouldn’t be either. Your methodologies and models have to be able to keep up with individuals’ changing behaviour
Businesses who are still dividing their customer base into only substantially sized, stable groups will never be able to build a truly personalised contact strategy. They will lose out to competitors who are able to forge deep relationships with their customers, personalising at every touchpoint.
Clinging on to these traditional methods of customer segmentation means there is little hope of gaining a useful understanding of a person’s needs. As Todd Yellin, VP of product at Netflix states, “Geography, age, and gender? We put that in the garbage heap”. Just like Todd, at Big Data for Humans we believe that customers should be grouped by their commonalities and their needs from you as a business. We apply cutting-edge Network Theory to our clients’ customer data to build out statistically similar communities of customers which our clients can use to quickly and easily track down the biggest pockets of revenue to go after within their existing customer base.
When one-size-fits-all doesn’t fit at all
The problem continues when it comes to traditional customer segmentation models which typically use best-fit clustering techniques to push customers into a pre-defined number of segments. This is useful if all a business needs is an overarching snapshot of customer behaviour. What it absolutely won’t provide is the granular insight needed to support a personalised customer contact strategy.
Think about the last 3 times you went grocery shopping. Based on the products that you bought, which one of these five mutually exclusive ‘segments’ would you fit into?
I know that for me, that’s an impossible question to answer. Last week alone I was a Top-Up-Shopper’ (the day I bought just bread and milk), ‘a Student Staple’ (stocking up on snacks and wine for a party) and an ‘Artisan Fan’ (I bought fresh fruit, vegetables and imported cheese). Like all shoppers, my behaviour was personal and nuanced and impossible for a retailer to understand using a ‘best fit’ clustering model.
A segment such as ‘Artisan Fans’ uses traditional clustering techniques to find customers who are more likely to buy premium fresh produce and organic goods. However, within this overarching segment, there is huge diversity – like the two groups below.
The Caveman Dieter and Stock-Up Vegan are very different. Would a retailer want to communicate with them in the same way, offering them the same discount on a ready-to-eat organic chicken curry for instance, just because they belong to one overarching segment? If they want to deliver any meaningful return from their marketing spend, they definitely would not.
Segmentation models for the future
As we look forward to the new business year, retailers will reap the rewards if their customer segmentation model is fit for purpose – and for the future. This means revealing, not hiding unique behaviours to enable personalisation at every interaction, to every individual, every time. It means staying on top of customers’ ever-changing behaviour, using sophisticated data science and A.I. models to pinpoint the pockets of revenue that exist within their business that will drive increased customer spend. Customers understand that the data they provide businesses with is an asset, and they expect it to be used carefully to deliver an improved customer experience.
For those businesses that continue to apply methodologies established in the 90s to their customers, it’s worth remembering that many of their customers weren’t even born when these approaches were first defined! Students, graduates and young professionals of today have always had online shopping, they won’t remember a time before Google and Facebook and have probably never been lost without a map to hand. The companies who will thrive are those that can adapt to these changes, and are tuned in and ready for even bigger changes to come.
Written by Sarah Jarvis - Senior Solutions Consultant, Big Data for Humans
First Published: http://blog.bigdataforhumans.com/the-clustering-myth-why-modern-retailers-need-to-move-on-in-2018
Please login to comment.
Comments