Segmentation: Fail to plan, plan to fail
15 Oct 2018
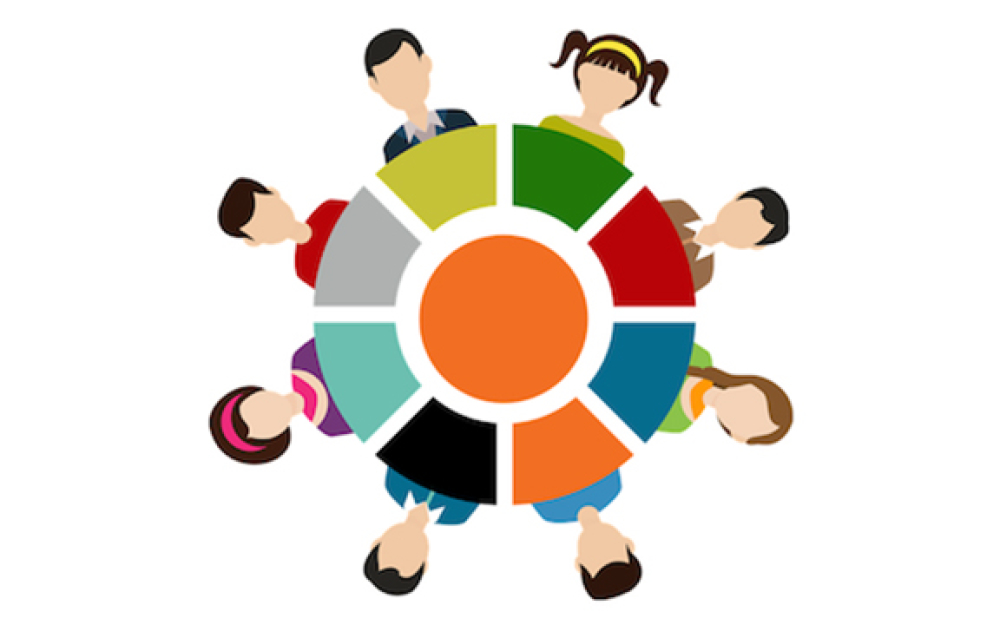
Unfortunately it is becoming increasingly usual to hear anecdotally of segmentation systems that are failing to perform to expectation.
Following the emergence of data science, deep learning and AI segmentation is right back on the marketing agenda, the appetite for which hasn’t been as voracious since the monumental success of Clubcard back in the late 90s.
This is good news as segmentation is an extremely powerful tool, but only if it is applied and executed in the right way.
The problem we have is that all too often organisations are putting the proverbial cart before the horse in their rush to create an all-singing, all-dancing competitive advantage panacea.
However, segmentation can only ever be as effective as the validity of the model on which it is built. The key to a good model is one with solid foundations. Without a clear objective a segmentation model is floundering in the dark, despite the seemingly endless patterns of data and insight it produces.
Not even the most experienced and wizard-like data scientist with access to a catalogue of data assets will be able to create an operationally sound segmentation system if they have no appreciation of end game. Consequently as with many strategies, the best policy is to start defining exactly what is it that the business wants to achieve.
What is it that the business can do to change behaviour on a person-by-person, channel-by-channel, date-by-date basis? Once this is established only then can segmentation be applied to the problem. It is here that the data science team can start layering up the segmentation hierarchy to create a multi-tiered, three dimensional model that can be deeply interrogated to find insightful patterns too complex for human comprehension.
By working backwards from the problem a brief can be drawn up and given to the data science team, against which they can design the model and determine all the necessary data sources.
The alternative is starting with the data and using all of it indiscriminately to try and create a crystal ball. But as we know the only certain thing about the future is uncertainty.
A good segmentation system is therefore not a future gazer but an engine that builds insights about an organisation’s customers and their behaviour and then interprets this understanding to predict their future needs so that it is possible to determine when individual consumers are in the market for specific products, what type of communications will result in a purchase and how to extend their overall lifetime value.
Any segmentation system that doesn’t do this is not delivering on expectations – but to be fair, the question that needs to be addressed is: has it been set up to fail in the first place?
For more information vist: www.outra.co.uk
Please login to comment.
Comments