Improving data for improved deliverability
22 Jun 2017
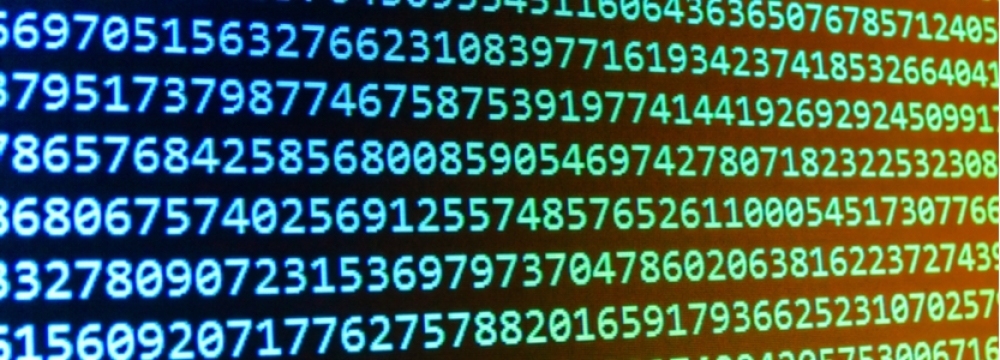
Regardless of your data methods, these suggestions should help across all data practices.
Let’s get this out the way, first of all, this may seem like email 101, but bad data is always bad data. If you are getting your data from the internet equivalent of ‘it fell off the back of a lorry’ or ‘I found it in a skip’, you cannot be surprised that your deliverability, engagement and overall profit are bad.
But what happens if you got it from some poor unsuspecting soul who forgot to uncheck a checkbox on a competition to win £500 worth of shopping vouchers? That data has opted in right? A shoe-in for your office supplies emails! Get those massive email conversions coming! What’s this? Someone has complained to Spamhaus and now you have been blacklisted. The major ESP’s have realised the poor engagement with your emails and stopped the majority of your traffic from delivering. But that data was opted in? Where are my conversions?! What’s happened?!
Now, this might be an extreme example but these types of things happen every day in the wonderful world of email marketing. Email has such a high return on investment, to the uninitiated the ‘must send more’ mentality seems to be a solution that would yield higher and higher returns, but the days of buying a list and ‘batch and blast’ or ‘spray and pray’ are numbered. GDPR will be the final nail in the coffin. And let’s be honest, it is for the best…
While even I admit not all purchased data is created equal, we would not recommend using purchased data.
On the flipside, you may have a database full of lovely organic and pure data. It is all nice and snug in your CRM/eCommerce system, you are very happy with it and so you should be. Here is the bad news: email churn is very, very real and it is affecting your results right now.
If you ask someone how many email addresses they have had in the last few years, you could have some surprising numbers thrown at you. In the early 2000’s Return Path said that 30% of all email address became invalid in a year, so using that modest figure that means 30% of all of your new signups this year are already dead.
The 3rd side to the mystical coin (has to be mystical to have 3 sides…) is you have a mixture of the both…
Now if you are reading this, you may have 5,000/10,000/500,000 or 20 million email addresses in a database and some of those could be as old as that Return Path article itself. That might seem like a terrifying prospect, but do not worry, we have a plan. It is time for some basic date-based filters and segments.
Again, these may be different depending on your customer lifecycles, but the principles should remain the same and can be adapted to many different date based fields (the last date of purchase/attended event etc). I would like you to have a think about how you could use these practices to help your own data, get in the inbox and most importantly, convert.
Try and keep purchased and organic data separate, or work out a way to separate them. Seeing how your purchased data performs will give you any indicators of how it is working for you, if at all. Low results on any purchased data should be met with utmost scrutiny.
While what is detailed below is not a one size fits all approach, it will give you a basis for trying your own engagement-based data response.
Sample data plan:
- Bin everyone who has never opened ever. These are subscribers who are not helping your cause. They could be dead emails, they could be temporary addresses. Why are these still in the database? You may want to set a parameter so new signups are not targeted, but that is down to you understanding your customer base and when an email address stops becoming a ‘new sign up’.
- Looking at the rest of your data and setting some engagement filters. Set one for ‘has not opened in a year’. This should remove a large chunk of your unengaged subscribers from the list and we can deal with these guys later.
- Split the rest of your list up into bite sized engagement chunks. For example 3/6/9/12 months. Then you know you have 4 different stages of engaged customers.
So after we have applied these filters, we now have no customers who have never engaged left (hooray) and we have 4 time-based engagement filters on the list. Because these are filters are of one main content list, people will move their way throughout these filters over your time emailing them. For example, someone who is in your 9-month chunk may jump into your 3-month chunk but may drop into the 12-month chunk.
Sample Sending Strategy:
-
- 3 months – These guys are your top subscribers, send them everything.
- 6 Months – Like the 3 months, you can be far more liberal with the sending here.
- 9 Months – Back off on these guys a little. Send higher offers or key brand building.
- Over a year – Minimal volume but more aggressive offers and brand building. Pull them into your other segments.
If you are attached to your data a little more than others create an 18-month or 24-month segment and send them only a few of your biggest announcements or sales. But between you and me more pragmatic types, send to them once a year max and send it slow… Or to you real best practice trailblazers – get rid of them forever.
Keen to learn more about deliverability, and how it can affect your email campaigns? Visit our Deliverability Knowledge Hub.
Please login to comment.
Comments