How Machine Learning Improves Customer Journey Mapping
03 May 2016
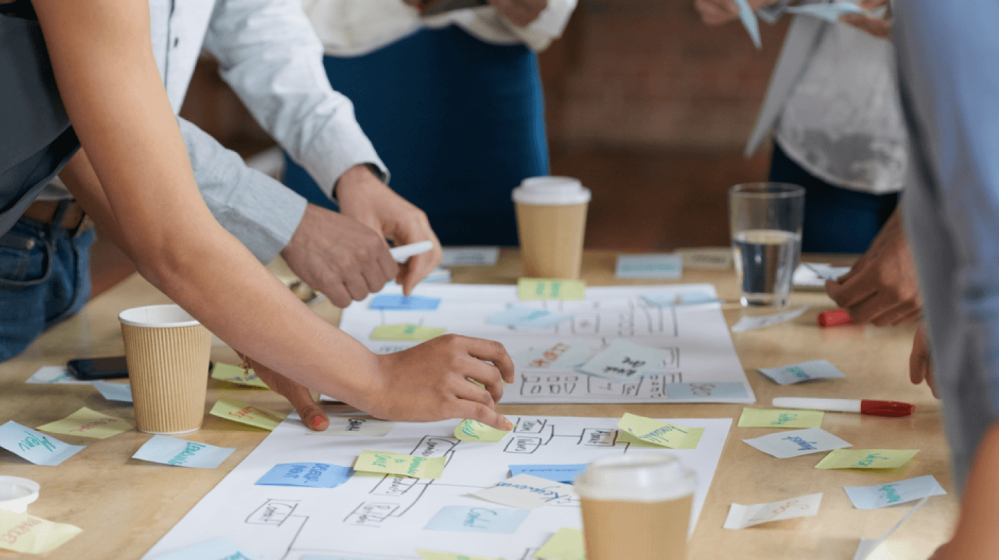
by Ankesh Anupam
Senior Consultant, Wipro Digital
In the digital world, business revolves around the customer.
Organizations are transforming from the traditional business model to a customer-centric and personalized offering model.
Customer experience has become the priority for businesses — the key to differentiating themselves from their competitors. By improving the journey across the stages of customer engagement, they can create more personalized offerings and ultimately drive higher conversions.
According to Gartner, in the next three years, 60 percent of digital commerce analytics investments will be spent on customer journey analytics.
Customers today have a number of choices in front of them — and organizations are still struggling to provide consistent, personalized and easy experiences. To provide personalized experience, organizations need to identify the customer’s needs and preferences. Eventually, they will be able to use that data to predict customer behavior.
Creating Accurate Customer Personas
In the past, various predictive methods used proven statistical and psychological models.
Leveraging these models, customers would be assigned different static personas, which would then be attached to a corresponding reference journey.
The reference journey would map to personalized offerings that would (hopefully) tie back to the customer’s classification. To better understand the process, take a look at the image, right. It demonstrates how the persona identification takes the customer’s preferences, need and the context into consideration when assigning a persona.
However, in reality, the customer’s interaction at different touchpoints is never linear. Instead, multiple options are usually available, and the customer often follows different personas’ paths at different stages in the entire journey.
For example, the diagram below shows the different personas for various customers at different stages of the journey:
Identifying the right persona at each stage of the customer’s journey and predicting the next behavior is not a trivial problem to solve, and involves processing a large volume of heterogeneous data from various sources and channels providing contextual information (like buying habits, attitude, feeling, emotion, social sentiment, timing, location, compelling reason, goals etc.) in real-time.
To achieve the “personalized” or “individualized” treatment, the persona also needs to be created at a micro level and having too few broad persona categories may not be sufficient.
The conventional way of processing the data using predefined rules or program and correlating and analyzing is no longer sufficient because of such huge complexity.
Advent of Machine Learning
Machine learning, a branch of artificial intelligence (AI), can perform and process tasks without being explicitly programmed based on the continuous learning using flexible, iterative and adaptive model, providing a foundation for solving the problem mentioned above.
With the increase of processing capability these days, machine learning clustering algorithms can be used to identify the patterns in the customer data from different sources, and can help classify a customer behavior to a matching persona in real-time.
Supervised vs. Unsupervised Learning Systems
To provide the best solution, organizations can use different types of machine learning clustering algorithms. The various clustering algorithms fall under either “supervised” learning system or “unsupervised” learning systems.
In supervised learning, the model is first trained from the existing data in a guided and supervised manner, where a controlled set of customer’s training data are categorized into a particular “persona” on certain matching attributes.
The similarity between the target customer’s attribute and the one in the training set will help in classifying the customer’s persona in a particular cluster. The similarity matching can happen on as many attributes as possible and the outcome will be dependent of the training data set’s quality and iterations of learning.
“Unsupervised” learning algorithms helps in identifying the pattern in the data without any knowledge. This type of algorithms increase the complexity, but prove handy when the attributes of the “persona” are multivariate and not easy to classify or cluster.
Both types of algorithms can learn on their own with the help of the data to adjust the model or business rules and tune it with the self-learning and adaptive capability to get best-fit model.
Take Customer Experience to the Next Level
The problem of customer’s intent identification is no trivial issue and with the limits on technology solutions, the identification process used to be static, making lots of assumptions and generating an unrealistic outcome.
However, the advent of technologies like big data and real-time stream processing, coupled with machine learning to analyze the large chunk of heterogeneous data from varying sources, helps in uncovering the dynamicity of customer’s “persona” with accuracy and when it is needed to act, disrupting the entire customer journey mapping.
Now, organizations can plan the interaction with the customer in the journey and fulfill the expectations, realistically bringing the entire customer experience to the next level.
Originally published on CMS Wire.
Please login to comment.
Comments